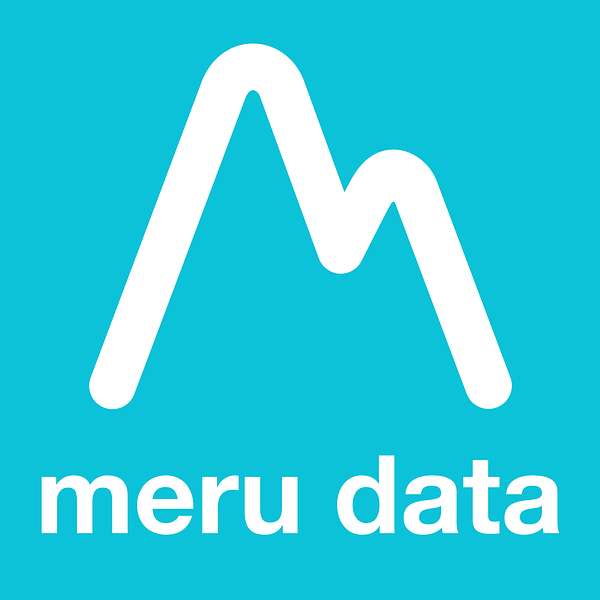
Meru Data's Podcast
There is tremendous value to simplification. To quote Steve Jobs, “Simple can be harder than complex: You have to work hard to get your thinking clean to make it simple. But it is worth it in the end because once you get there, you can move mountains." In this series, we explore how people and companies achieve simplification.
Meru Data's Podcast
Simplify for Success - Conversation with Katharina Koerner
Katharina Koerner was on #SimplifyforSuccess, a podcast series presented by Meru Data and hosted by Priya Keshav.
The conversation between the two privacy professionals centered around Responsible AI governance, including different risks associated with AI, strategies to identify these risks, possible approaches to building in-house AI governance structures, and picking an appropriate framework to operationalize AI within companies.
They also touched on the EU AI act and other regulations that covered AI and the intersection of privacy and AI while discussing different reports on AI, like Datagrai Survey.
Thank you to Fesliyan Studios for the background music.
*Views and opinions expressed by guests do not necessarily reflect the view of Meru Data.*
Priya Keshav
Hello everyone. Welcome to our podcast around simplifying for success. Simplification requires discipline and clarity of thought. This is not often easy in today's rapid-paced work environment. We've invited a few colleagues and data and information governance based to share their strategies and approaches for simplification. Hi, Katharina, how are you?
Katharina Koerner
I'm pretty good. Thank you for inviting me. How are you, Priya?
Priya Keshav
I am great and welcome to our podcast on Simplify for Success, would you? I don't think you require an introduction, but would you mind introducing yourself to our audience?
Katharina Koerner
Of course, not! It's a pleasure. So, uh, yeah. My name is Katharina Koerner in Austrian, German. I am based out of San Jose, CA, Silicon Valley. My background is I have a PhD in EU law and various certifications in information security, privacy, privacy engineering and machine learning. I was to see all of an international education group for five years before I moved to Silicon Valley from Austria, and I worked in the Austrian public service, also for 10 years in the Ministry for the Interior as well as the Ministry for Foreign Affairs. My last role was at the IAPP. That's the International Association for Privacy Professionals. I was the Principal Researcher, Technology and focused on privacy engineering, technology regulation and AI research. And currently I'm involved with the Tech Diplomacy network that's also here in Silicon Valley and the Tech Diplomacy network was founded to foster collaboration and dialogue between the diplomatic community, civil society, and the tech industry. And as a side project, I want to mention, my latest endeavor is that I founded the AI Education Network, which is a volunteer initiative to help Kid, 12 and high school students, as well as teachers without understanding of AI, to also actively participate in the discussions around AI opportunities and challenges. So, I hope this will grow somehow and become bigger.
Priya Keshav
Well, that's actually great. So, I want to kind of digress, but we'll get back to our podcast in a second. I have kids and I was just talking to someone about how I'm here. These school districts have taken different views. There are many school districts that have sort of said they would ban AI and others who have said they will accept AI as a reality and try to incorporate AI into their curriculum and so I have teenage daughters and younger kids. So, I'm very concerned about this idea of kids using AI as well as kids’ data being an AI and safe usage of AI. So, to the extent that I can participate in any way, I'd be more than happy to kind of join your initiative and contribute, so.
Katharina Koerner
Thank you so much. I will come back to you. Thank you.
Priya Keshav
So, we are going to talk about AI governance today. As the adoption of AI grows, there is an increasing recognition that enabling trustworthy AI is very, very important. AI, however, is a very broad term and can cover a broad range of use cases, right? AI issues and choices will differ based on sector, based on the type of problem it's trying to solve. For example, the consequences of bias or error will differ based on whether its usage is for healthcare versus criminal justice versus retail.
What type of approach would you recommend taking to understand AI use cases, to classify them for governance, especially if most of our audience tend to be within corporations looking at AI governance. Some of them may have a mature program, some of them would be just starting to think about building an AI governance structure in house. So, would you take a one-size-fits-all approach to governance, or would it be more nuanced? What are your thoughts around it?
Katharina Koerner
So, my thoughts around that are in general apart from model risk management that has been a regulatory requirement for banks since 2008, I personally do not see that you have different organizational governance approaches for AI which would require to classify AI systems from the onset on, so that then you have different processes depending on such a classification. But we will come to talk about the NIST AI risk management framework I think a little bit later in more detail, and there you have to have the option to create different use case risk profiles for different applications through the frameworks, functions, categories and subcategories. So, for example you can create AI risk management framework hiring profile or their housing profile and also take into account your business objectives, legal requirements for that specific area, your resources, or your risk management priorities.
So, I think from the onset on in general, I have not personally seen different approaches for the governance structure, but for the different applications you would then have different risk profiles. Does that answer your question?
Priya Keshav
I think I wanted to maybe drill down a little bit further, right? Like for example, sometimes AI usage can be also not so obvious. You know, as you sort of start making an inventory of all the use cases from within your company. I was just thinking about Zoom as a good example. The video conference software just recently changed their terms of service to allow for harvesting of private user meeting data, and of course, they clarified that they were not going to do that. But it sounded off an alarm. But you sort of see this often where, as you look at contracts, many contracts probably have hidden clauses in them, which allows for usage of data for various research and development or other purposes that have AI use cases. And so, you know, as you sort of start thinking about AI and AI governance, you sort of have this set of use cases that are maybe obvious based on the organization and then, as you sort of explore you realize there's so much more.
You know, AI has been a term that has been overused. And sometimes that might be just a marketing strategy, sometimes there is actually AI behind the scenes and also as you look at the definition of AI, one of the things that keep in mind also, you know, as you look at regulations. We'll probably talk about the regulations later, but some of them define AI more broadly. So, you know, one of the challenges that I see is also the fact that just understanding how much exposure to you have to AI is a challenge as you start building the governance framework. I don't know if you agree or not, but that's my view.
Katharina Koerner
Yeah, I mean, one thought I have here is first, what do we mean by AI governance? Because in my perception, people refer to different things. So, some people refer to regulations, some refer to international law even, some refer to responsible AI as a governance guideline within organizations. But I totally agree with you. What do we even mean, and I'm not talking about the legal definitions now, like what is AI today? What do we mean by AI adoption? Like, what are the use cases that are really out there, and everything. They looked into the data breaks report on AI adoption, they surveyed more than 9000 global customers. That's quite a bit. And the report highlighted that business intelligence is currently still leading segment in the data in the AI markets. So, that means processes and tools that enable organizations to analyze and present data for informed decision making. So, kind of the classic use case I would say. So, visualizations report, dashboards, query data on demand, data mining, predictive analysis, performance score cards, that's still what is out there when people refer to AI.
And what I also found interesting is that in regards to LLM's, because it's such a big topic nowadays. They're kind of diverging information that I come across at least. So, for example, the data bricks report said that LLMs are adopted at a rapid pace, with the number of companies using SaaS LLM APIs, so, for example, to access services like ChatGPT with the growth of 1310% since last November or between November and May, actually. While other reports such as, for example, I can also recommend looking at this one, it's very nice and interesting by Pretty base called Beyond the Buzz, a look at large language models in production. They didn't service so many, but 150 enterprises, still in 29 countries and maybe the various countries where, like the reason why the data differs so much. They came to the finding that only 1% of companies said they have more than two LLMs in production and only 13% said they have one or two LLMs in production, and companies are planning to use commercial LLMs in real world use cases, to that question, almost 80% said no, due to privacy concerns, due to the cost, due to the lack of customization. And those companies who were using LLMs, it was primarily for summarization for content creation and for creating structured tables from unstructured data like customer emails, website content or PDFs. So that's to the point like what is actually, where is AI or machine learning actually used.
Priya Keshav
Makes sense, right? And that brings me to another topic, which is how do you identify and manage reputational, relational, regulatory, and operational risks around AI? And you know, I think that engineers and data scientists especially know more about where AI is used and how it's used. But they're not trained to look at risks, identify risks, and be able to sort of quantify risk. And you have the risk management team who are trained in identifying risk, but they may not be, you know, they may not understand AI. They may not fully understand the technology behind the scenes and sometimes they're never looked in. So how do you build a strategy around identifying risk? Because that would be a critical step in managing and governing AI. So, what are your recommendations around it?
Katharina Koerner
So, I think if I start talking about the least risk management framework right now, it would maybe be a little bit too abstract. So, maybe I start by referring to the report I conducted last year. So last year I compiled the report for the IAPP with FTI Consulting and we surveyed how many organizations of the ones we talked to, already held responsible AI governance guidelines in place and how do they try to operationalize them. And, what we saw here? I'm, I'm jumping around a little bit, but I want to refer to exactly what you said.
One challenge that was named by a lot of companies is exactly what you said that so many domains are involved. Legal, privacy, engineering, data science, business functions and all those teams which are great subject matter experts in their fields. They often have limited cross domain knowledge and that leads to siloed perspectives that only cover partial aspects of responsible AI. And that's why we're seeing in those companies who already put their guidelines into practice, they very, very often start with working groups or AI ethics support. I mean, it doesn't really matter how you call it, but somewhere where different domains, various, you know, subject matter experts come together and address very basic questions, and maybe even start with just one use case. Like, what do we mean by various terms? What is? What does even mean privacy to you? But because when we look at the term privacy, for example, privacy in privacy enhancing technologies or in differential privacy means something completely else. Or it's just a part of what it means to a legal person, right? So even, like very basic terms mean different things to different people. So, this is why it's so important that I think we, we start with coming together, looking at maybe just one use case and starting to ask our questions like what do those responsible AI principles that I think we will talk about later in a bit more depth, mean to us? What does non bias mean to us? Do we have ideas? Do we already have approaches? How to address it? Does it mean for us that we have to have diverse teams as well? Or do we currently just address it with, I don't know, statistical bias? Is this what we mean by bias?
So, I think just coming together and starting to talk is the very first step. And of course, you can like have a really, really good and come up with a really, really good risk management, assistance. And here I would recommend for a couple of reasons to really have a look at.
Priya Keshav
I agree, and I think I was just looking at Mackenzie's paper and identifying risks, and some of the things that they were suggesting. You mentioned them too like using a framework. So, we've talked about NIST a few times today and we'll probably talk a little bit more about the NIST framework and other frameworks that are out there. But they talk about use it as a way to sort of guide you to identify it is in a more methodical fashion, but also kind of refer to databases of past risks. So, to see patterns of what others have seen as issues with a particular type of AI, and they've made some suggestions around REC teaming too, which is challenge people to, or encourage people to identify not so obvious risks. So those are actually great, great ideas that I thought would be worth mentioning. But what you said in terms of cross functional approach would be critical. So, we've been talking about the NIST framework, so let's talk about AI governance framework and obviously that would be very foundational, right to pick a framework. So, there are multiple frameworks out there. You know, how do you pick one? Is there, you know, does it matter which one you pick? And, you know, how do you sort of adopt a framework as you operationalize AI within the organization?
We could just focus on this too, but if you had thoughts on other frameworks or picking, that would be great.
Katharina Koerner
Yeah, I do. But I mean, I think that a lot of frameworks, I mean, for me personally, the term framework is already not because you're using it, just in general. It can mean so many different things and it means different things to different people. So. To some people it GDPR is a framework. Maybe it's a framework, so I would just like to start by going over the basics like what is responsible AI or what is ethical AI and what is trustworthy AI? So, for me it's all the same and we have various frameworks and resources that lead to a really very clear profile actually, what responsible AI is.
And here I think I will mention some frameworks that you're referring to so. Responsible AI can seem like a fluffy term, but I think by now it has a clear profile as I said, and it is a set of good governance guidelines that are composed of a set of common principles, and this set of common principles very typically includes privacy, data governance, accountability, auditability, robustness, security, transparency, explain-ability, interpretability, you know, some frameworks use those terms, others use others. But in an overview, it's like they're very, very, very much overlapping. Then they have of course fairness and non-discrimination, human oversight and promotion of human values. And there are many resources for those responsible AI principles, starting from international organizations such as UNESCO's recommendation on the ethics of AI. Then we have the Council of Europe's report towards regulation of AI systems. It's a little bit older, but still, we have the OECD AI Principles. We have the ethics guidelines for trustworthy AI by the high level expert group at the European Commission. Then we have a very similar set of principles which were published by nation states such as China's ethical guidelines for the use of AI, or the US Vito's blueprint for an AI Bill of Rights.
Then we have a similar set of principles of frameworks by industry initiatives, together with academia and nonprofits. For example, by that partnership on AI or the Global partnership for AI and beyond that, we find really almost countless self-regulatory initiatives for companies’ frameworks which is super useful to take a look at. I mean, I'm really a big fan of Microsoft responsible AI high standards. They also have so many additional documents and playbooks to understand how this can work in practice. We have Google's responsive AI principals, Salesforce trusted AI principles, Facebook's 5 pillars of responsible AI, and then additionally, we have those standardization bodies such as ISO, IEC, IEEE and NIST which also offer guidelines.
And all of them, like I said, overlap in the definitions of principles, so there's a very solid understanding of responsible AI, at least on this policy and governance level. And if I would pick one, I would totally go with NIST because. I do not only think, but I have read that, the US, despite or in the light or while, however you want to say, there is no comprehensive AI regulation on a federal level, it really wants to move forward or deny it, to commit it to advise the White House on AI. They also really advise or suggest to use NIST AI Risk management framework, to internationalize it, to push it and I think it even is complementary to the EU AI Act, which I think we will also address a little bit later. So, I think it's a really good framework and a really good approach to build your AI risk management system within your organization.
Priya Keshav
So would you kind of want to talk a little bit about Why you like NIST or what parts of NIST makes sense? Maybe that's a bad question. But I was just wondering if you had any further thoughts on why you picked NIST or why you would prefer to kind of use NIST.
Katharina Koerner
I mean we do know the NIST’s Cybersecurity risk management framework is like all PA and not French speaking, so it's like usually mentioned in one breath with ISO 27001 or 27000 Family. So, I think that's a good example of how NIST does already have internationally recognized frameworks. And I do not only think, I mean, I do think it is a, it can be a challenge to really embrace or understand where NIST is going. But it has also this playbook on the side where it needs recommends, are questions you can ask that are very concrete to your teams, to everyone involved. NIST also mentions who should get involved at which stage the AI life cycle. And I mean, I think it's not easy. It's not easily accessible, but I think it's not only a very good sign, or like you position yourself very good as a very responsible organization by looking at it, by saying we used it. I mean, you don't have to copy paste anything. You can really just use what you want. It's a voluntary framework. But I think it will go a long way and we already see various legislations such, for example in California, we have, we have a draft bill for AI, and it already refers explicitly to those four functions that NIST is mentioning: govern map, measure and manage. So, it's already introduced in proposed to US state level bills. I think that's a sign that its relevance in regulating AI systems and mitigating risks of AI will increase.
And if you really take the time and look at it, you can really see how those categories that I mentioned govern: so, establish good governance as a foundation. Then map: contextualize your AI risk by categorizing the AI systems, understanding the context. Then measure: analyze and monitor the risk. Use quantitative or qualitative tools manage it, allocate resources, establish monitoring. So, all those things are in this framework and those categories are then divided into subcategories and then into specific actions. So, you can, really as an organization, have a very structured approach to risk management and the framework does encourage you as an organization to customize it so that it aligns with your unique context, with your objective, with your legal requirements, with your risk management priorities, and that you can have a tailored use case risk profile as I mentioned before. So, I think everything you need to know is in this framework, plus the playbook that comes along with it. I think it's super practical and you have everything in one place and it's just also very good for your posture, AI risk management, posture to reassure stakeholders that you are aware of NIST’s work and that you have built on it.
Priya Keshav
So, you mentioned principles and talked about Microsoft's principles. You may see many organizations adopting a principle-based approach to govern AI governance, where maybe they have publicly talked about the principles that would govern their AI usage. But let's say an organization starts with that, which is defining some of the guiding principles for AI. How do you sort of move from principles to actual actions? Or how would you operationalize it internally as you build your program?
Katharina Koerner
So, I think the operationalization of these systems is very much discussed, and religiously so, because we have a vast ecosystem of research and practice that is being involved. And this ecosystem necessarily works on very complex solutions to complex problems which again then makes it a bit tricky to relate their findings to what we really aim for, making AI trustworthy for the end user, or translating the necessarily complex findings to legal requirements. But, nevertheless, to start with the principles is already something that is not yet done by every company.
In this report I mentioned before from last year, I found that 60% of organizations surveyed already had published ethical AI guidelines, meaning playbook standards, commitments, declarations internally or externally, and 40% of organizations without guidelines were planning to develop them. But it is true that as the survey continued, it became evident that organizations struggled to acquire sustainable tools to address risks in practice associated with AI and clear pathways how to put those responsible AI principles into practice.
One challenge is, as we mentioned before, that so many domains are involved. Another challenge is that leadership support might be inadequate. There might be deficient resources, improper knowledge management, absence of incentives, or lack of team diversity. And then we're also facing this information overload and unclear legal and technical compliance requirements. But nevertheless, we also found that, kind of the good news is, that organizations are actively building expertise in the fields of law, data science, AI governance to address AI related challenges. And that we have role models or best practice examples that we can somehow model or that we can, yeah, take as leading examples. So, where there are companywide programs to responsible practices very often starting with developing taxonomies. Like what do we even mean by those terms? By providing training and education, by maybe having a response by champions programs so that in different business functions you have someone who is really interested in it and who says I want to know what those principles mean for our business unit, and I want to talk with my colleagues about it, I want to raise awareness so that you can foster community, peer groups and start to embed AI ethics as a cultural practice.
And then we also saw very clearly, that very often those AI ethics boards or working groups are set up and that processes that you already have in place, like for example your privacy program, that this is leveraged for your AI governance. So, for example, the privacy impact assessments are leveraged for AI impact assessments. Questions are added. There are also some suggestions out there with how you can use that. But it's true that in the end, the data scientist or the machine learning engineer is the one who has to really put a lot of should put a lot of those principles into practice. And this is just not a simple task. And sometimes it's even impossible, I would say. So, we still have this, I think, responsible AI operationalization gap at the very end, because it's also not easy to find resources how you should even do that. Like what is a bias detection method or a bias prevention method that everyone should know of. What is it?
It's not that I get like so many answers to this question when I asked this question like recently like two or three days ago, I posted something on LinkedIn. I reposted, actually I went through the NIST document on bias again, towards standard for addressing bias in AI, something like that the report is called. It's very good. It emphasizes that it's a social, technological challenge. And I don't know it got like 700 likes. And then I also asked in the comments section, can you tell me or educate each other, what are some common bias detection tools or methods that everyone should know of, especially legal people but also for example, because I believe it's good when the Governance, AI governance function sits under the sea salt, but the answers are scarce. I mean, there's group fairness methods, but still it's not easy for anyone I think to really know on top of the minds how to address those things.
Priya Keshav
Now you bring up a good point. Like I said, right, the data scientists understand the technology, but I think how to identify risk, how to quantify the risk? How do you mitigate it? I think we have a long way to go.
Katharina Koerner
I would agree.
Priya Keshav
Moving on, let's talk a little bit about the AI regulation. And we can't talk about AI regulation without talking about the EU AI act. The world's most comprehensive or the first comprehensive AI law. It looks like MEP's adopted the parliament negotiating position on AI act and now they will start. Each country will start beginning to work on the final form of the law, right? So, what are your thoughts around the EU AI act?
Katharina Koerner
Yes, as we for sure have all heard as, you say, the EU is currently in the process of developing the EU AI Act and also the EU AI liability directive, as part of its data strategy, The EU AI is currently in the trialogue negotiations. That means that's the EU decision making process that involves the three legislative bodies. That's the European Commission, the European Council, that is representing the Member States and the European Parliament which represents the people. We might see the finalized AI act, a political agreement on the ACT on a text reached by hopefully late 2023, so this year. And then we might see a finalized AI act adopted early next year. And then after 18 to 24 months transition period, the AI act would come into effect. So that could be late 2025 or early 2026.
So, and what's the content like? What should we know about it and what is already like kind of not under discussion anymore so. In all circumstances, the new rules will have extra territorial effect, such as the GDPR. So it will apply to providers of AI systems established within the EU or in a third country placing an AI system on the EU market. And it will apply to the users of the AI system, not the end user, but the user who is, you know, a company that is using the AI system of the provider that is located in EU. And it will also apply to providers and users of AI systems located in a third country where the output produced by those systems is used in the EU.
Then the Act will include a definition of AI systems which is currently still being discussed. And the classification system based on a risk-based approach, I think we have heard of this, like almost all of us have heard of this, that they will, that the act aims to prohibit AI systems which have like unacceptable risks. It would authorize high risk systems only under specific requirements and low risk systems would be subject to some transparency obligations.
Then the EU Member States will have to designate competent authorities. Spain has just come up with its authority not waiting for the EU act will be passed, I think 2 weeks ago or last week it announced a new AI authority. And it will also include a national supervisor authority to oversee the implementation. And the Member States are required to take appropriate actions to restrict or even withdraw the high-risk AI system from the market if it doesn't comply with the regulation, with administrative fines being up to 30 million or 6% of the total worldwide annual turnover. And this EU AI will then complement it by the liability directive. That's not so far progress, but still, this liability directive will address civil claims for damages in case of harms due to AI systems. And I think we should also not forget that we do already have laws in the EU, especially the GDPR, the General Data Protection Regulation which applies to all AI systems that process personal data, and we do have the NIST directive that regulates the ICE systems used in critical infrastructure and the medical device regulation relating to AI based medical devices. So that's like kind of the overview of the EU.
Priya Keshav
So you also have, I mean obviously not that there aren't, you know, no activity on the low level, but you see a lot of activity at the state level and we'll talk a little bit about the intersection of privacy and AI before we end. But you know as you know, 10 states included AI as part of the larger consumer privacy law that will be going into effect sometime soon. And many states have proposed bills, or they may have appointed a task force to investigate AI or made some kind of a resolution or a statement around AI to both express concern as well as kind of indicate this is an area of interest. There is a lot of activity happening in the US around AI as well, right?
Katharina Koerner
Yeah, totally. I mean, so while we do not see this comprehensive horizontal federal level, AI dropped yet and I personally do not think, but I'm not like a policy person in so much, but I do not think that the federal privacy law will pass, but. It would have required or it would require AI impact assessment. That's true. So, the US does have a lot of regulation of AI already, I think it's just there's a lack of enforcement resources. So currently the US approach is more tailored to specific sectors. And it's dispersed across various federal agencies with for sure, the FTC, the Federal Trade Commission, taking center stage, which uses its authority to protect consumers against unfair and deceptive practices. Also, for the realm of AI, that was, for example, demonstrated just recently when they opened the investigation into OpenAI’s ChatGPT in July, it was like pages of pages of questions.
Then and also the FTC has published several posts that are really easy to read about its expectations, how companies have to build and deal with their AI for consumer protection to, I don't know, avoid discriminatory impacts. And it also announced to take action against companies that make claims about the AI that are not substantiated or deploy the AI before taking steps to assess and mitigate risks. We have seen the FTC already requiring firms to destroy algorithms, so this algorithmic disgorgement or other work products that were trained on data that should not have been collected. So it's pretty serious to see what the FTC is doing and we should really be aware of this.
And besides the FTC, the Equal Employment Opportunity Commission, the EEOC is very, very active in the field and since a long time already. The EEOC can impose transparency requirements for AI demand, a non-AI alternative for individuals with disabilities and enforce non-discrimination in AI hiring. And then we also have the Consumer Financial Protection Bureau, the CFPB. The CFPB mandates explanations for credit denials from AI systems, and also has the potential to enforce nondiscrimination requirements. And then there's also, and those are four bodies actually released the statement just a few weeks ago. They're really keen to use their power to look what companies are doing. So, the 4th one is the Department of Justice Civil Rights division. And this division enforces constitutional provisions in federal statutes prohibiting again discrimination across many facets of life, like including education, criminal justice system, employment, housing, lending, voting also related to AI, and automated systems.
And yes, I can go on because as you said, apart from these approaches on the federal level, we have many U.S. states that show interest in regulating AI. For example, California has introduced AB 331. That's a law specifically targeting automated decision tools, and will, if it's going to be passed, a mandate developers and users to submit annual impact assessments. Then we have this super prominent example, the New York City local Law 144, which took effect on July 5th. This law requires that automated employment decision tools undergo annual bias audit by an independent auditor, and that the summary of those audits will be publicly shared. And we have similar bills already been proposed in New Jersey, California and Washington DC. Yeah. And then we have not yet talked about, you know, privacy laws and proposed bills for AI in other states because there are plenty.
Priya Keshav
Let's talk about privacy, right? Like, obviously, you know, when you look at AI regulations and where AI regulation is headed, it's sort of paralleled privacy, and we just talked about forming a cross functional committee. We talked about doing impact assessments. We talked about a lot of things that sort of very similar to what we do in privacy. So, while there are a lot of overlaps between privacy and AI, AI is also a, you know a completely distinct area with risks that go beyond privacy. It's not something that is just, you know, a complete overlap right like so. But what are your thoughts around the intersection of privacy and AI and yeah. And that, how that probably plays a role in defining how AI might be governed within organizations?
Katharina Koerner
So yeah, that's I totally agree with you. It's not only about privacy, although your question is about privacy. I heard you. So, we have those issues that are widely discussed, and for many good reasons, copyright infringement bias, incorrect outputs, deep fakes, public safety and so on. But we do have complex privacy risks to individuals, organizations, and society, especially when we look at, I mean the latest developments of generative AI, we should all be very aware that that globally accepted privacy principles such as data quality, purpose, specification, use limitation, individual participation of course also applied to all systems processing personal data, including training algorithms and generative AI.
And I do see a couple of main problems here. I think the most challenging, maybe one, yeah, maybe perhaps one of the most challenging privacy questions in machine learning in general: How to exercise individual data privacy rights? So, one such important and globally accepted right is the right to be forgotten, and that allows individuals to request the company delete their personal information. And while it is comparatively easy to remove data from databases, it is very difficult to delete data from a machine learning model and doing so may undermine the utility of the model itself.
Then another issue is transparency, so that's of course also a responsible AI principle. I would also argue that most of responsible AI principles that we talked about before are already covered by privacy regulation actually, but nevertheless, let's talk about privacy so. There is broad regulatory consensus in privacy that information needs to be provided to individuals about how the data is collected, how it's processed, and that information should be accessible and sufficiently detailed to somehow empower them in the exercise of their rights. And in the US, by the way, the Federal Trade Commission has already issued guidelines for data progress to advise them how to be transparent about data collection usage practices and those requirements are just not easy to live up to, when we're trying to translate or anticipate algorithmic predictions.
And then I think there's also this issue of data scraping which somehow overlaps with privacy because this is such a complex topic that is not regulated in the same way globally. So, for example, in the US, US state privacy regulations such as the CPA or the CPRA. So, the California Consumer Privacy Act and which was amended by the California Privacy Rights Act, they exclude from their scope, information that a business has reasonable, has had a reasonable basis to believe, is lawfully made available to the general public. Unless, for example, the website terms of use prohibited data extraction, if it's assumed that the general public can access the information, then there's probably, I mean there's not a general prohibition of web scraping.
On the other side, in the EU under the GDPR, an explicit legal basis is also required for public data. If you fail to protect personal data from scraping, that also infringes upon the obligation of the website providers to protect user data and might put individuals at risk.
Priya Keshav
You raise a lot of points, right? I mean we can talk about, actually this entire topic and just intersection of privacy and AI for an hour, on its own. So, but thank you so much. Before we sort of conclude though, do you have any closing back?
Katharina Koerner
I do hope that, I mean the research out there is so immense, so impressive. So cool. I mean, there's so many. There are so many, many people, idealists or just, you know, bright minds who try to find solutions to problems that are currently unsolved and that really excites me. So, for example, there is this nascent research area called machine un-learning.
They are trying to solve the problem of deleting data from machine learning models or I don't know methods like reinforcement learning with human feedback that can support more accurate model training or we have privacy enhancing technologies such as differential privacy, or people searching, researching how deep duplication of training data could contribute to solution. So, I'm a big fan of all those smart researchers out there, and I'm hoping that industry will pick up their ideas and implement them so we can move forward with innovation because I love the things we can use today.
I would not have wanted to live in Italy or like ChatGPT was not available for a while. I do want to use those tools and I do think we will find solutions to combine, you know, privacy and protection with new technology. And so that's my hope. And my optimistic outlook for the future.
Priya Keshav
You couldn't possibly end the show on a more optimistic note than that. But yeah, I do agree that I don't think, you know the question is to stop using these technologies or to stop innovating. The question is how to kind of do it responsibly, where we're able to kind of progress innovation, progress usage, and also protect the privacy of individuals, protect other rights, you know, that individuals have so. Thank you so much for joining us, Katharina, it's always a pleasure to talk to you.
Katharina Koerner
Thank you so much for inviting me, Priya, and thank you for keeping us informed with your podcast.