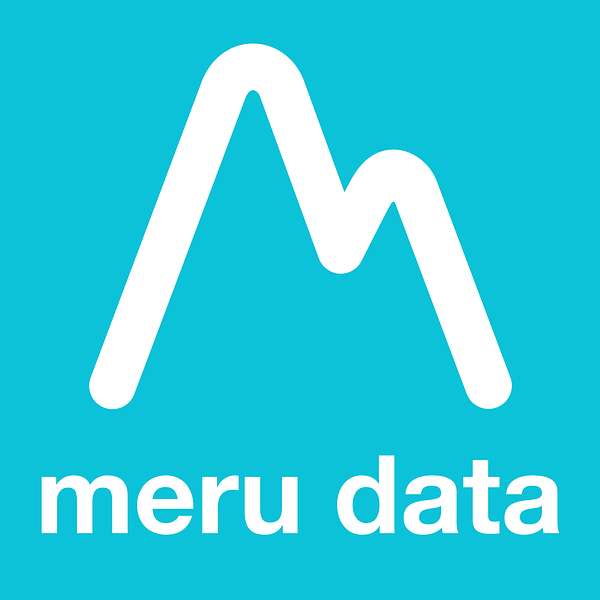
Meru Data's Podcast
There is tremendous value to simplification. To quote Steve Jobs, “Simple can be harder than complex: You have to work hard to get your thinking clean to make it simple. But it is worth it in the end because once you get there, you can move mountains." In this series, we explore how people and companies achieve simplification.
Meru Data's Podcast
Webinar Audio - How to Incorporate AI Governance into Your Privacy Program
The EU AI Act is close to being finalized, and California just released the rules for automated decision-making that will regulate the PI used by AI is protected and prevent biases.
Given this unprecedented importance given to AI in the world of privacy, companies are called to ethically and effectively incorporate AI into their privacy programs so that their AI systems and practices avoid noncompliance with the upcoming regulations.
Brought to you by Priya Keshav, CEO of Meru Data, this webinar is geared towards a brighter future, inculcating the importance of ethical AI practices to reshape the business landscape.
Some of the many topics covered in this webinar:
- Current regulations around AI.
- Privacy best practices for AI and Machine Learning
- How to build on existing privacy programs to incorporate AI
- Ethical and responsible use of AI
Elevate your business and transform your legacy by watching our exclusive webinar.
This webinar is approved by the IAPP - International Association of Privacy Professionals (IAPP) for Continuing Privacy Education (CPE) Credits.
Tune into our Webinar Audio with privacy experts Priya Keshav as they discussed these questions. The experts also talked about the significant changes in the privacy world, citing different laws introduced by the lawmakers.
Priya Keshav
Good afternoon, everyone. My name is Priya Kashif and I'm one of the founders and CEO of Maru Data. Welcome to our webinar on how to incorporate AI governance into your privacy program. AI is everywhere. The AI systems process large amounts of personal information as companies ramp up the use of AI. As privacy professionals, if you are wondering how to incorporate privacy into AI design. Who's responsible for ensuring risks around AI are managed? Then we will discuss the multifaceted issues around AI and privacy within AI. Examine the potential risks that are associated with AI implementation, challenges in governing AI, and strategies for incorporating AI governance into Your Privacy program will also discuss some actionable steps and strategies that you can take now, as you sort of maybe build out the AI governance program. As I mentioned previously, my name is Chris Kashyap. I'm one of the founders and CEO of metadata. We're a company that is focused on building solutions to simplify privacy programs for customers worldwide. We help in identifying and managing privacy and security risks around data. And obviously, as AI usage increases, AI governance becomes part of that process as well. Prior to metadata, I was the managing director at KPMG's Forensic Technology Practice in the southwest U.S. I have more than 20 years of consulting experience in risk management, information governance, cyber crime response, technology investigations, and privacy. So before we get started around AI governance, let's talk about what is AI, because that's something that we need to understand. The use of AI is predicted to grow by 25% every year, and AI is supposed to contribute about 15 trillion to the global economy by 2030 two thirds of the US executives surveyed by KPMG last year. They surveyed about 225 US based executives, and they felt that I will have a high or an extremely high impact on their organization over the next 3 to 5 years, and areas of impact included employee productivity, looking at innovation within the company and customer success. As you can see, all three areas probably touch um, personal information because customers and employees are impacted. Um, consistency, standardization and responsible use of AI will be key to a successful implementation of AI within any company. The 45% of the executive survey felt that, um, I will have a very big impact on the trust and reputation of the company if there wasn't any proper risk management practices in place. What is AI? Um. It is can be defined at a very simple level. And again there are so many definitions. So the first step to AI governance would be to align on a definition of AI within your company. But AI is a machine performing some type of cognitive function. It could be inference, it could be reasoning, it could be learning or teaching. It could be interacting with an environment like a chatbot. Um, it can help in problem solving. It can help in productivity enhancements. It can help in marketing and profiling. Um, understanding customer sentiment, fraud detection, uh, in exercising creativity like generation of images, videos, etc.. So, um, yeah, it has a number of use cases. Um, and these use cases are increasing every day. So you're finding new uses, um, of AI. And so it's important to sometimes look under the hood. Um, what we may consider as normal function may be something that is performed by a AI behind the scenes. And many times, as privacy professionals, when we start looking at data flows, it's not that, um, surprising to find that, um, there is data that is going to a model in some fashion or the other, um, and some component of the overall system, uh, probably is performed by an AI. Um, there are so many versions of AI and of course terminology. So it's important for us to understand a little bit about some of the terminologies that are commonly used. And again, this is not an exhaustive list. Um, but, you know, a narrow AI, it's important to understand the types of AI. A narrow AI is an AI that is built for to perform a specific task with a larger accuracy. So it's more, uh, targeted in its objective. Um, and but as a generative AI, um, consumes a large amount of text. Um, they are LM is probably the most common example of a generative AI. But there are other types of generative AIS as well. So not all generative AI is an LMS, but most LMS are probably generative. Is a generative AI. Um, and then discriminative AI is used to distinguish two types of outputs. Um, so again it's a type of narrow AI. Um, and then you can have a generative AI that can perform, uh, you know, variety of different tasks. So it's not built for a specific purpose. Um, you've also probably heard of the term self-aware AI. At this point, it's only a theory, but, um, there's definitely a lot of speculation and conversations about how self I already self-aware I already exists or we're pretty close to getting there. The definition of AI in various regulations, sometimes it's broader than just some of. So it's important to also understand that you look at, you know, AI governance and your jurisdiction. And when you identify all the jurisdictions that apply to you, it's important to understand the definitions that are relevant. To you as you consider AI governance program. I just want to pause at this moment and um, if you have questions, feel free to kind of add questions or comments to the Q&A. Um, I'll be answering them as we go. Um, and to the extent that you have additional information that might be useful, feel free to share that as well. And I'll be sharing that with the others. So um, and I've just got two examples, um, of definitions from a regulation standpoint. The EU AI actress was approved um recently and and as you can see, the definition slightly is different from how California defines AI versus the UI act. But for various reasons, most of the regulations broadly define, um, AI, and that is to make sure that the definitions, um, are not going to get outdated at some point because AI is evolving so much that, um, as we start defining AI the way we see, I might be very different in five years. And so they don't want the definitions to become obsolete. So that is partly the intention. And the other intention is also to kind of bring a lot of different systems within the scope of the regulation. So as I won't go into too much depth about, you know, in analyzing these definitions, but we'll talk about it briefly. Um, so UI defines AI as a machine based system that operate with varying levels of autonomy. So autonomy is the key word here. Um, and also that may exhibit adaptive ness after deployment. Um, in complying with either explicit or implicit objectives, infers from the input it receives and generate outputs as predictions, recommendations or decisions. So to some extent there is an emphasis on the type of output that might be relevant. You know, whereas uh, California defines a you know, they don't use the word AI, they use the word automated decision making technology, uh, to indicate any system, software or process. So it might not even be a system um, included. One derives from machine learning and statistics. So they go broader than AI based systems and other data processing or artificial intelligence. So they kind of just make it much broader than AI that processes personal information and uses computation as a whole or part of a system to make executive execute decision to facilitate human decision making. So here the biggest emphasis is on anything that kind of facilitates some sort of an automated decision making is considered to be within scope, um, for the regulation. So it's important to kind of think about the definitions. And um, you may want to align on what would fall under or what constitutes AI within the organization. And that might be based on again, maybe again, your jurisdiction. It could also be based on use cases that you see, um, and what you think is relevant because, um, again, the purpose of your governance might be broader than just meeting the requirement. For particular regulation. It could be to kind of look at other, you know, biases and other issues that are on AI and govern it broadly. So every organization is different. The objectives might be different. Um, so your definition is definitely going to vary based on those objectives. Um, and this slide is just to kind of show that how many use cases exist. Somebody once mentioned that, you know, once you start looking at AI specifically, especially as you start data mapping and inventorying all the AI, usage within the company, one thing you'll realize is, as you sort of start asking questions, you might realize how prolific AI already is within your company. Um, and that might be because, you know, like I said, as you start analyzing the workflows and look under the hood, you may find you might find different use cases, and some of them might be, you know, more. Um, as you start, we'll talk a little bit about how we classify those. And so some of them may be high risk and more relevant, and others may be, you know, use cases that are more benign, but it's still are they are still I use cases and it needs to be recognized. So if you just look at obviously Jenny you know is a everybody talks about ChatGPT. Um, but if you look at uh gen I use cases, there are so many use cases for Jen I and this is by no means an exhaustive list is just starting point, but it can help you in marketing content creation, so you may be using it to generate your brochures. You may be using it to generate articles. Um, and we've known about the fact that I can hallucinate. So as you sort of use it for content generation, it's important to think about, you know, what parts of it is real and what parts of it may be made up. Um, it might be used in conversation analytics, you know, looking at customer sentiments, um, grouping certain types of, uh, customer conversations, things like that. Uh, it might be used for fraud detection. It could be used as a virtual try ons if you are a retail, uh, retailer and maybe you can kind of there are use cases now on the ability to kind of try a certain dress or look at designs online, Um, or if it's a furniture, you could possibly look at how it fits in within your house. So there are so many use cases, um, that I base around for retailers. Um, similarly, it could be they could, it might be managing your portfolio. Um, it might be part of, uh, clinical trials, drug testing, design optimization, whether the design is external or internal, uh, it might help with supply chain traceability. Um. Chat box is another very common example of I use cases. It might be adding, uh transcript. Uh, transcribing your conversations, um, maybe taking notes, summarizing, uh, documents, filtering, um, helping with reporting, um, maybe in content cleanup, like data quality, uh, cleanup. Um, it might be helping in personalizing. Certain content, like repetitive information, might be automatically displayed or entered by AI, as opposed to asking humans to do that manually. It might be part of your search and recommendation engine. It might be helping in software, not just code generation, but in quality control. It might be helping in software documentation. So there's a lot of AI use cases now in helping with software documentation. I already mentioned summarization. So as you start looking at, um, use cases, you may find many of the products. As you start looking under the hood, there is use cases. Um, it might also be translation is another example. So it's important to have look at those use cases and have some awareness about the use case. So you can identify and start inventorying and tracking them in your data map. Um there are some regulations in place already, but this area is evolving. Utah just passed one, and there are a number of, uh, movements in this area. So, you know, even a month from now, this might look a lot different. Uh, but some of the regulations that are existing today and we'll only briefly touch on them today. But I see a question. So, you know, I was asked a question how I would differentiate between rule based systems versus AI systems. Um, so there are nuances, rule based systems, depending on how the algorithm was developed. Again, it depends. There's a lot of, uh, details. Right. But I would say rule based systems are probably going to be limited in its ability to kind of do certain things because it's a it's more of a if then, um, and it's more, you know, the way it's built is more, uh, what I would say restricted in scope compared to a I based, you know, if it is built on top of, let's say, a DNI. Um, so as you consider the risk and as we consider the scope of, you know, the, your governance, uh, how you sort of evaluate the rule based system versus how you, um, evaluate a system that was built on top of AI will be very different. Well, maybe talk a little bit about it as we kind of look at governance. Um, so it's important to know how or what type of AI it is. And that's one of the reasons why I covered the different types of AI. And you know, it's obviously not exhaustive, but it's important to yes, it's it's AI or automated decision making tool. Um, again, it depends on how you define it. Um, but also what is the technology is built on, what is the type of AI we're talking about? Because how you sort of look at risk for different types of AI. Um, there might be some things that are common and some things are different and it's important to, uh, to know the differences. So, um, the UI just passed. We also have various privacy regulations and, um, you know, pretty much all of them apply to I use cases as well. Um, there is an executive order that only applies to, uh, government organizations, but it can influence private entities as well. Um, you have, uh, the law that was passed in New York, uh, around automated. A meditation tool and I'm not covering mostly covering just EU and US. Um, and then you just recently passed a law. And so let's talk a little bit about California as part of its, um, you know, CcpA, you know, it's now just introduce uh, rules around management of, um, automated decision making technology. And um, some of the so they the rules focus on only specific areas. So they don't kind of look at governance of all AI, but more around the type of decisions made by AI. So if it's used for financial lending, housing, insurance purposes, if it's used for educational enrollment or opportunity to get education, if it's used within the criminal justice system, if it's used for employment, independent contracting opportunities or for compensation. Determination of compensation for employees if it's used within healthcare, um, if it's part of essential goods or services, or for profiling or behavioral advertising. Um, and of course, to the extent that personal information is used in AI. The general rules around privacy still apply. But for the most part, if AI is used in one of those use cases that we just discussed, that's when some of the rules around admit apply. And obviously it's still not finalized. Um, but important to look at what is in it because as you sort of think about your governance program, you want to incorporate, um, some of what is required under the rules, um, you know, into your program so that you're, you're ready when it's finalized. Um, as I talked about, if and it's not if pretty much most systems, um, that we talked about like because the emphasis is on, um, customer support and customer interactions and also employee productivity and innovation are the three major focus areas. And there are also studies that indicate that places where you see AI deployed a lot is in data analytics. And most of the data analytics in organizations tend to be customer oriented. So typically what you may find is that many of the use cases that are on AI, it is not everything, but a lot of use cases around I probably has or uses personal information. Um, sometimes it's evidence. And that's one of the I mean, as privacy professionals, when you start asking questions, uh, it's not uncommon for people to say, okay, there is no PII in my data. So first of all, the definition of PII is very narrow, uh, in most people's mind. And so they kind of don't think about personal information is broader than PII. And they also sometimes, you know, as you feed raw data, you don't, you know, clearly understand that there's a lot of personal information. Sometimes that is part of AI training as well as AI, um, input and output that needs to be considered. So um, so sometimes it's not very evident at the beginning that personal information is involved, but very likely a lot of the systems has personal information that is being fed to it. And um, as you look at privacy, you know, issues. Is obviously all of the things that we talk about every day, you know, in terms of data collection, processing, storage of sensitive data, personal information, as well as data minimization, your privacy rights, access rights, your collection rights, your erasure rights. Um, if it's used for profiling and behavioral advertising, then your opt out, right? All of that may apply. And to the extent that it's using personal information, especially depending on the use cases, there might be a requirement, um, for doing privacy impact assessments. Um, and so which means you probably have to do a document, your impact assessment. And it's also important to make sure that if there are the data is being used for training, if the data is used for within AI, there's clear notice and disclosure. So all of the things that we talk about for personal information, because it's kind of part of the AI system, um, they all apply to AI as well. And um, you know, if New York would be a special, very narrow use case where if employers or employment agencies are using automated decision making tool to kind of do, uh, any type of recruitment decisions, then they must do a bias audit. Um, so if you are a provider or if you're using systems that are being that was purchased from a third party, then important that you have completed and a bias audit and you've looked at it and that needs to be part of your vendor management process. If you are procuring the tool. And this law is already been in effect January 1st, 2023 and is enforceable since July. So hopefully most of you have already had something in place to manage this. But if not, it's something that you need to consider. If you have employees in New York, um, EU, I act. We can talk the entire, uh, webinar and more about the AI act, but you know it. I wanted to use this as an opportunity to talk about, you know, not all AI is the same, right? So it's important to once you start inventorying the AI, like I said, there are so many use cases and you might you'll find AI part of everything. Um, it's important to think in terms of how they apply. An EU kind of AI act provides a methodology. This might not be what you might want to use, but it's something to consider. Um, but it's important to know again if you are developing it. So which means you are a provider. So you are it's something that is developed by you internally or if you are consuming it so which means you have purchased it as part of the tool and it was developed by someone else. So obviously your uh, how you sort of approach governance will be different, um, depending on whether you're a provider or a consumer. Um, and the other thing to kind of think about is as you sort of, as a number of use cases increase and as you look at all the different use cases, it's important to look at how to classify that. Right? So you're not all of them are the same. So obviously in the case of you some use cases are prohibited. That might not be the case everywhere. Um, but they are, you know, another way to look at it as they are very high. High risk use cases. So if you're using them for those kinds of use cases, then you probably want to really look at how you're governing it. And then you have your high risk use cases that are still relevant and important. And then you may have some medium and what I would call low risk use cases. So how you approach governance has to be risk based. And the risk might be based on how you know many things, right. It can be based on, uh, use cases. So what is how the data is being used and for what purpose. But it could also be based on type of data that is being, um, used by AI and the volume and other factors. So, um, most people, I mean, at least a common way to classify them seems to be more around, um, you know, what is it being used for and the data that are being part of it. So it's important to have a good understanding of those two things about your AI, which is what is the use case and what type of data is being used to train the eye to, to, as well as as input and output for the eye. So let's talk about overlap because this our webinar is about how to incorporate AI governance into your privacy program. So FTI and IIP did a survey about two years ago um, to look at what are companies doing to sort of incorporate AI governance. Um, what they found was that more than 50% of the organizations building new AI governance approaches are building responsible AI governance on top of existing mature privacy programs. Um, and I will talk a little bit about why. Um, so if you are thinking about adding AI governance to your privacy program, you're not alone because there's at least 50% of those who have surveyed who are doing exactly the same thing. Um, some of the big things is responsible AI principles like explainability, fairness, security and accountability are also requirements in the privacy regulation. Um, organizations that clearly explain governance models for responsible AI. Um, see, there is a lot of close collaboration needed for governance. And we talked you know, we talked about some of those things. Right. So, um, you know, as you start looking at, um, documenting AI based systems, you'll find maybe 70 or 80% of the use cases is using some kind of personal information. So which essentially means that privacy is going to be in scope. Right. Um, you're also going to notice that what they are evaluating against, um, you know, in terms of the principles like I have listed here, the responsible AI principles along side by side with the GDPR principles. Um, you know, you can see a lot of common, um, you know, it's similar. It's not exactly the same, but there is a lot of similarity around how you look at AI versus how you look at, uh, privacy. But it's important to know as you look at, you know, the fact that they're similar, it's also important to understand that the scope of AI slightly expands beyond privacy. And we'll talk about. So it's important to not completely ignore that. And if you consume it completely into your privacy program and only look at privacy impact and only look at personal information impact, then you may be missing out on. Some other things that needs to be considered as well. Um, so it's important to keep that in mind. Um, but a lot of work there is overlap between privacy and AI governance. Um, and so the question is, would it make sense to sort of blend it into your privacy program or, you know, are you going to kind of look at it as somebody you collaborate with very closely, like you do with security? So even now there are many organizations that have sort of merged security and privacy. Um, so and then there are many that will say that privacy separate. So some of this depends on the company. Depends on your resources, depends on funding. Um, but things to consider as you kind of look at AI governance programs. Um what is AI governance. What are you supposed to do when you kind of look at AI governance? And, um, I've oversimplified it, but if you look at the, um, this framework, um, they talk about mapping. So first understanding what type of AI, uh, exists, making an inventory including shadow deployments with AI. You may also find many shadow use cases of people sort of using things to summarize their documents, asking questions to ChatGPT. So a number of employment, I mean, deployments might be relevant and good use cases, but you may also apply in a lot of shadow deployments where AI is not so visible, but nevertheless it's there. And so Also, looking at your cross-functional user community that both promotes the collaboration and openness of openness with respect to using I promote best practices, you know, bringing questions back to the team. So it's important to kind of build that culture within the organization. Uh, because I governance, um, is a new area just like privacy is. And it's going to, you know, it's evolving, um, a lot as we speak. So it's important to, um, have that two way or multiple directional communication back and forth so you're able to sort of evolve as things evolve around you. The other part is to manage. So obviously there are requirements around, um, understanding risks around AI, um, prioritizing those risks and taking a risk based approach to solving some of them, and then communicating with your top management around IRS and and the governance program so they are aware and are able to support you with your efforts. Um, and then the governance part of it, in terms of developing an AI policy, um, making sure your privacy policies are obviously reflecting the AI usage, training, um, your team on best practices and on use of AI. And if you have a policy and some guidelines that you want to enforce and communicating those, um, you know, looking at the types of controls that you want to have in place. And then of course, the measurement piece, which is to see how well your program is working. So but um, as you kind of look at your privacy governance, uh, a lot of this is probably already in place. I mean, you're constantly measuring, reporting on your privacy program. Your privacy policy is not your AI policy, but. There's a lot of, um, you know, you need the data. You need to develop your AI policy might be similar to the data. You need to kind of make the disclosures you need for your privacy policy. Uh, your training can. And if you already have the, uh, a way to kind of make sure that your, you know, um, develop and implement training for privacy, a lot of that can be leveraged. So the content might be slightly different. Your privacy impact assessment can be, um, extended to also include AI. So like I said, the risks around I might be broader than privacy. So it's important to understand that it's not exactly the privacy impact assessment you may want to do. You may have to look at other risks as well. But um, it's probably the percent there. And then you have to add the other 40% to it. Um, and there are challenges, uh, you know, from an implementation of, uh, your DSR, um, uh, obviously providing disclosures might be easy, but, um, deleting data from a model API was used for training. Uh, obviously it can be very difficult. So looking at some of those aspects and as you think about controls might be important. And of course data mapping, um, is an integral part of the privacy program. So extending the data map to include AI, uh is not going to be a big effort on your end. So let's talk a little bit about data risk. So obviously, um, data is going to be data feeds, the AI. And a lot of things that are related to privacy is around data and AI risk might also be around data, but um, it's important to kind of look beyond that as well. Um, so obviously you're going to consider as part of security, um, what would happen if the data is breached. But in the case of a normal system, you're probably just going to think in terms of just, you know, what happens if the data is disclosed or if there is unauthorized access. But, um, you know, in the case of AI, there can be other risks where I can react in a different way, which might cause more harm, uh, or to a person, um, you know, whether it's your employee or your customer or a third party. Um, and the data usage, uh, expectations, disclosure and constant consent is kind of very similar to what we kind of think about in privacy, so that that overlaps. Um, but you have to think about biases. You have to think about misinformation, uh, hallucinations, IP because the data could be trained, the fundamental model that AI is built around, um, could be trained using, uh, data that might not belong to the company, um, or might not have the proper consent for training that data. So, um, so it's important to understand those aspects. Um, so you can kind of look at um, as you think about Iris, you can think of them as people related risks, organization related risks and ecosystem related risk. Um, people risks are probably going to overlap a lot with privacy. Um, your organization risk, um, is um, might be might overlap a little bit with your security. Um, function. Um, but the ecosystem and other risks, uh, might be something that might not be covered within your privacy or your security program, and you might have to, um, engage with other stakeholders or allow other stakeholders within your organization to participate to even, you know, understand and surface some of those risks because, um, one of the biggest problems, just like, you know, being able to look at your scope by defining AI as it's relevant to your organization is key. Um, as you kind of look at your privacy impact assessments or AI risk assessment. Understanding the risk is going to be very important. Uh, and it's not very obvious. Um, sometimes the risk can be much more complex and will require, uh, people with different skill sets, people with different experiences to kind of come together, to kind of be able to provide a more come up with a more comprehensive list of risks. Um, but, um, a lot of them, I mean, if you think about if you understand the data, um, usage, um, your what is being used to train the base model. Um, the data that is used to as input, is it going back to kind of improve the base model, or is it just used for generating the output? What does the output look like now? As you start focusing on the data aspect, you've probably covered a big portion of the risk. Um, and this is by no means, um, you know, an exhaustive list of questions. Um, you know, there's a lot of nuance to understanding, um, what type of data is being used. But at a, at a very minimum, you should be asking questions like what was used? What was the source of the data to train the I was there, you know, how did that data get collected? You know, was it what did we have the right you know, does it include personal information? Do we have consent even if it doesn't include personal information? Where did that data come from? Because, um, most um models require a large amount of data. And it's a challenge to kind of build that kind of data set. So if it belongs to someone else and, um, you know, it probably was not, um, developed with, uh, there might be IP issues to consider as well. And then, um, you know, not just whether you had consent or rights to that data, but what kind of data was it? You know, how do you know that it is a representative? Um, you know, um, it represents your entire population. There are no biases. There are no built in, um, problems that will obviously get extrapolated as you consider your model. So being able to understand the base and that's again a huge difference. You brought up this question of rule based versus um, you know, AI that is developed on, you know, a uh, foundation model like generative AI. Uh, you know, it's important to understand. What are you looking at? And so how you evaluate that base model might be different depending on how it was developed. Um, the um, obviously, um, then if there's personal information involved and sensitive personal information involved, then, uh, you know, there are more considerations in terms of what is the Pi, um, and what is the sensitive Pi and whether you really need it for, uh, decision making or for training and obviously looking at protecting that information, obtaining consent, being able to opt out, being able to delete, being able to provide a copy of it, you know, and all of those things are huge challenges, uh, when it comes to AI, because we don't currently have a good way to delete data from a model. Um, there's a lot of research, uh, but we're not quite there. And so, um, so it's something to think about as well. And, uh, and the amount of data being used. Um, so one of the things as you kind of think about, um, data, it's important to understand that there's a lot of research being done around risk. And, uh, what it's easy for somebody to pull the training data by, um, hacking the model. So, um, so sometimes if you use personal information for training, you have to look at not just the fact that, um, you know, when there is a breach, is there a possibility of somebody pulling, uh, all of the sensitive information or the personal information that was used for training and retrieving it? Um, and what are the consequences of that happening? Um, if the AI system is relaxed, then, um, what are some of the issues of misinformation or hallucination with respect to personal information that can affect your, um, that can be harmful to a person. So there's there's a lot of considerations to think about. And sometimes it's more than just, uh, it requires more than just the privacy team to kind of be able to come up with a list of risks. Um, and it might not be as straightforward as you start looking at it because, um, Pia is, uh, doing a Pia for privacy on a regular system is pretty challenging on its own. Um, and especially as you consider use cases like behavioral advertising and profiling. Um, you know, we, uh, we still have a lot of challenges in understanding, um, real time bidding, how, uh, the data moves and what is really personal information, what is identifiable, how is how does cookie track things out? Pixel tracks things and now you add an AI to it. It just makes it much more complex. Um, and so it's important to have more than, um, you know, bring on as many being the right stakeholders to be able to help you with the risk management process. This is actually, um, this was pulled, um, from a Carnegie Mellon paper. Um, and we will definitely share the presentation with you. So, um, you'll be able to see the source of that. Um, so, but I. It's just used as an example to show, um, how much privacy risk exists and the type of privacy risk that exists in, um, an AI model. And so it's um, oftentimes I come across conversations where, you know, it's easy for us to sort of overlook, um, you know, the AI, I'd like to use, um, recommendation systems as an example. Um, you know, it's when you look at it, it's easy to sort of think about recommendation systems as all you're doing is showing, um, you know, more music or more episodes, um, based on past listening history. And if you're not actually profiling or if you're not inferring anything from that beyond just making more recommendations of similar, um, content, um, maybe the risks are low, but actually, if you start looking at it, um, you know, there's a lot of research and study that has happened with respect to recommendation systems that it tends to sort of show you more and more, especially, um, you know, teenagers who listen to, um, maybe they are depressed and they listen to a certain type of content. The recommendation engine feeds more and more of, um, you know, similar type of content, uh, which, um, can affect the well-being of that individual. Um, or maybe, you know, it's, uh, some of this content is, um, around politics or certain topics that, you know, if you're fed similar content, it can influence your, uh, thinking process so that it can cause real harm to the society or to the person. So it's it's hard to understand some of those risks at a, at a federal level. So it's very important to spend time, um, looking at risks. I see one more question. Um, the yeah, it's an excellent chart. So I'm happy to share the paper. Um, that was presented, um, that this is, uh, this is not mine. So I'm happy to share the source of this, uh, paper, uh, with the audience after the webinar today. So, um, these are examples you're probably already familiar with. And there are many, many, many such examples. But Cambridge Analytica is a great example of how, you know, you provide some answers that you think is innocuous, related to a pop quiz in Facebook, but then it's used to sort of do a psychological profile for users. Um, similarly, Strava used um, heat map or released their heat map. They thought that it's de-identified data, but what they didn't realize is that when you have large volumes of data, it's easy to expose the the the locations of military bases. It's an inadvertent disclosure, but many times this is something to consider with AI systems. You may think that you don't have personal information or the person is not identifiable. Uh, Google has a as a game, and I've played it. It's actually a very fun game. Um, Google doesn't have um, so the game, you know, you can use technology to. Identify the location of a particular place. Um, you know, a street location. Um, which for a human, it's quite difficult. You can kind of maybe predict the country, but to be able to say, um, you know, find the precise location is much harder. But as I, um, because it can, it has access, especially if you're looking at AI that is built out of a foundational model. It has access to a lot of other information that might take a completely irrelevant piece of information that you and I may not be able to process, but but be able to use that to provide more accurate predictions of your location. And similar things can happen with people as well. Um, it might be able to correlate large amounts of data to be able to identify you, uh, from information that you may think is not identifiable. And so it's kind of important to keep that in mind as you sort of think about personal information. And I um, Flickr another example where millions of photos from Flickr to train its facial recognition software. Um, and obviously there was no consent. Um, and that was considered a secondary use harm. And the images initially shared on Flickr, um, were not for the purposes of developing the AI. Um, and you also see deepfakes. Um, you know, sometimes all you need is a, uh, photograph or a couple of photographs and a little bit of voice to create an authentic deepfake. Um, so, um, it's it's important to, uh, sometimes think about some of these use cases as you sort of think about risk, right? Like you may say, okay, there are, you know, it has maybe one image of a person what can happen with that? And it's a public image. Um, or there's a little bit of voice in there. So what is the impact of that? But all that, you know, a breach of just that one image and some voice, um, audio file can help someone build, uh, a deepfake, uh, which can be harmful to an individual. So it's important to understand that AI extrapolates problems that we already have, uh, but many, many times. And so risk as we understand today, is not what it will be. Um, you know, even a few years from now. So I, um, cut in state. So KPMG did, like I said, mentioned, um, did a study last year and they looked at AI governance. Uh, current state of AI governance. And they what the study found was that only 5% of the company surveyed. That was 225. Executives have a mature AI governance program in place. So, in other words, AI governance is new. We're all kind of in the beginning stages. Uh, it's evolving. Um, the regulations and laws or new or so yet to be, um, you know, finalized or approved, but it's coming. And 19% of the companies surveyed indicated. They are in the process of implementing an AI governance program. So only 20% 5% have AI and another 20%, around 20% sort of are in in the process of developing something. So a large amount of uh, 75% do not have anything in place yet. Um, and that might be because there isn't a clear understanding of the use cases that are for AI. It could be that, you know, they fall under the 27% that people who feels that AI usage is minimal. Um, and that could be real where the AI usage is actually minimal, or it could be just a lack of understanding. Um, I remember, um, seven years ago walking into many organizations, um, talking to CIOs and, and they'll say, oh, we don't have anything. Um, you know, in the cloud, for example. And then you will find like around 40, 50% of the systems are in the cloud, except not the clear understanding or, you know, we have very limited personal information and it's in this place, whereas you understand that personal information is, you know, everywhere. So, um, many the problem is most don't have good data map. And uh, when you don't have a good data map, you don't have a good understanding of your data. And the same is true with AI. So if you don't have a good data map and if you're not asking questions about, you know, the technology behind the scenes, the data flows to the various components of the system. You know, sometimes the obvious use case for the system might not be AI related, but as you dig deeper, you understand that there is an AI component because there's no module or certain features that you know are AI enabled. So being able to kind of look under the hood, being able to inventory can make a big difference. And so as you sort of do that, obviously one thing that you may realize is that one, there's a lack of understanding. Second. There is nobody who is, you know, um, holding the fort. Nobody's managing AI governance. There's no one responsible. And as you saw with the E&P FDI survey, um, privacy teams are picking up the slack. Um, they are owning the AI governance. It may be that privacy will own AI. Maybe that privacy will be part of AI. Um, nobody knows what the future looks like, but, um, it's something to keep in mind as you kind of think about, um, how to manage Iris. So, um, if you're just starting, like many of the companies, um, there are some key things that you can do today. Um, obviously, this isn't an exhaustive list of everything you need to do. Uh, obviously it depends on the maturity of your AI governance program. Um, but the starting point would be to understand your AI usage. Um, so making sure your data map incorporates AI. Um. With. If you don't have a, you know, making sure your privacy policies reflect the use of AI and then if you if you're not quite there to develop a AI policy, at least developing some guidance or best practices might be a good starting point. Um, looking at cross-functional support, um, you know, building that either steering committee, you can call it a working group. But, uh, who are those people within the organization who needs to be involved in this process beyond just privacy, beyond maybe just security. Um, and what does that look like? And how do you start education and training? How do you start disseminating best practices or guidances? Um, how do you start getting people to ask questions, be have conversations with you? Um, can you incorporate AI into the Pia or your risk management process? At least, you know. um, to the extent that you can easily incorporate and then you can kind of think about how to identify roles and responsibilities for the broader risks that might not fall under the privacy umbrella. Um, maybe you take that on or maybe somebody else kind of, uh, supplements risk management process with, um, you know, uh, around those risks, um, looking at your data management practices, data governance practices, If you have data governance program in place, looking at how, you know, they can look at data quality, how they can look at auditability of that data, looking at your vendor management process to see if they are, um, the third party risk management process is asking questions about AI. Um, you know, can you incorporate data minimization? Um, the key is do you really need to use personal information for training or in any of the AI models. Can you limited? Can you eliminate it? Can you pseudonymous it? Can you anonymize it um, before it's used by AI? Um, and of course, if you have to use personal information in AI, then, um, looking at what how you will fulfill the de sa process. So these are again starting points. Um, as you sort of evolved evolves from here to a more mature program, you need to kind of think about, um, the AI framework that you would use. There are already many out there is honest or good, uh, good ones to kind of refer to. Um, if you're looking at how to, um, model threats or even identify threats, uh, I shared one paper, but there are other tools as well. So happy to provide some information on them if you're looking for one. Uh, but definitely there are there's a lot of, uh, good resources out there on AI threat modeling. So being able to kind of use those as you sort of look at your risk management process will be, uh, important. Um, can you recommend data governance privacy, an AI governance framework that can effectively integrate. Um, so I would say it depends on. If your organization is using this, it might make sense to think about this. Um, as at least for privacy, governance as well as for your AI governance. ISO can be another, uh, framework, uh, to rely on data governance. Do not a team typically follow um, a completely different framework? They don't usually go use ISO or nest. Um, so if you are trying to integrate with your data governance, but they're not dramatically different. I mean, fundamentally all all of these frameworks, you'll see that there's a lot of common things across them. So, um, if you have a data governance program in place and you want to integrate with them, um, I can I mean, I'm not sure who asked me that question, but I'm happy to kind of, um, um, I'm happy to kind of answer this offline, but there are ways to sort of look at how they integrate that with their data governance. So if you could send me, um, you know, a message with LinkedIn, I'm happy to respond to you offline. And, um, and I also saw a note saying that there's a lot of information being generated around AI governance. It's, um, it's something that everybody's thinking about. Uh, and obviously there's a lot of, uh, information because of that. Um, so if you are looking for resources, as this person mentioned, there won't be any shortage of resources. Uh, but sometimes too much can also be a, you know, good and a bad thing. Um, so if you're looking for advice on what are good resources to sort of focus on and those that sort of maybe are not, you know, um, or might be more professional, I'm happy to provide some feedback as well. So feel free to ping me, um, via LinkedIn on a respond. So, uh, according to Brookings Institute, um, an artificial I want to leave us with this, which is, as artificial intelligence evolves, it magnifies the ability to use personal information. And I talked about this in ways that are not imagined today. So we're yet to see the ramifications of AI and privacy. Um, and it can definitely intrude on our privacy interests by, um, you know, making personal information and analysis of personal information to new levels. Um, so it's important to start thinking about, you know, whether it's you drive AI governance or there is a separate AI governance function within your company, privacy needs to have a very big seat at the table. So, um, I'm not sure where you are, where you are driving AI governance or you're looking at having a large seat at the table. But as privacy professionals, it's important for you to understand. I understand AI governance, the trends in AI and best practices around AI, and hopefully this webinar sort of, uh, provided at least, um, foundation around some of those topics. Um, but like I said, I'm happy always to be a resource for anybody who wants or has questions. So feel free to reach out to me. Um, if you have questions, um, on LinkedIn and I'm happy to answer. Thank you.